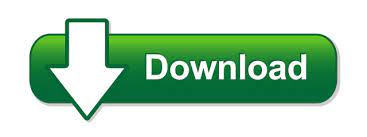
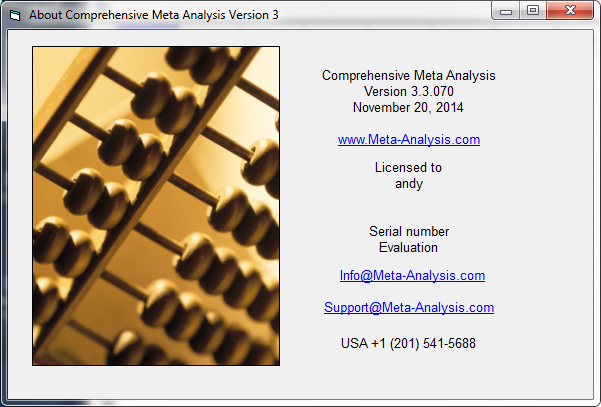
Therefore, the random-effects model is formulated as T i = μ + ε i + e i. The parametric effect sizes can be modeled as θ i = μ + ϵ i, ϵ i being the errors of the parameters around its mean, μ. In a random-effects model, it is assumed that the effect-size estimates, T i, estimate different population effect sizes, θ i, that is, T i = θ i + e i, and θ i pertains to a distribution of parametric effect sizes with mean μ and variance τ 2, usually called between-studies variance. Therefore, the effect-size estimates, T i, are also normally distributed with mean θ and sampling variance σ i 2, T i ∼ N(θ, σ i 2).
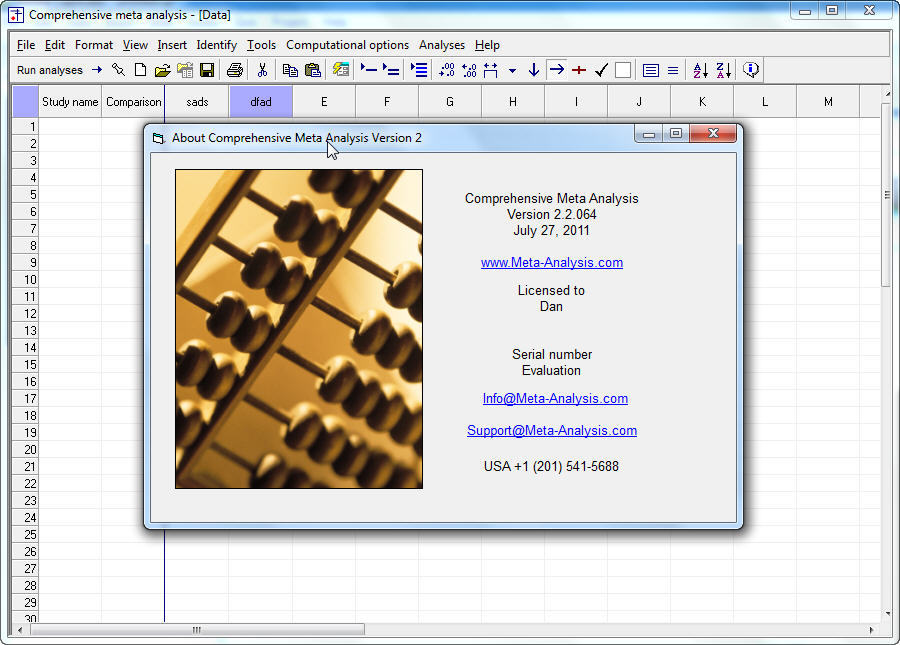
Thus, the model can be formulated as T i = θ + e i, the sampling errors, e i, being normally distributed with mean 0 and sampling variance σ i 2, e i ∼ N ( 0, σ i 2 ).
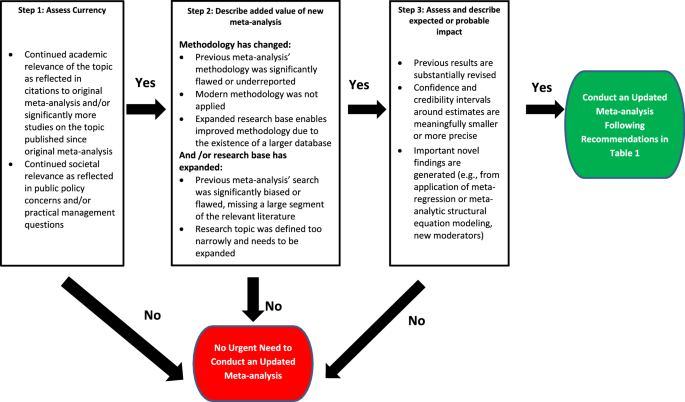
Suppose there are k independent empirical studies about a given topic and T i is the effect-size estimate obtained in the ith study (here T i refers to any of the different effect-size indices presented above, both from the d and the r families.) In a fixed-effects model, it is assumed that all of the effect-size estimates come from a population with a common parametric effect size, θ, and as a consequence the only error source is that produced by sampling error, e i. To accomplish the first purpose in a meta-analysis, that is, to calculate an average effect size, two statistical models can be assumed: the fixed- and the random-effects models. The main task of the statistical model is to establish the properties of the effect-size population from which the individual effect-size estimates have been selected. The statistical analyses in a meta-analysis are guided by a statistical model that must be previously assumed. Marín-Martínez, in International Encyclopedia of Education (Third Edition), 2010 Calculating an Average Effect Size
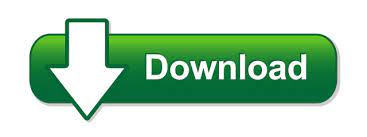